The question of the impact of generative AI and large language models on expert networks has been considered for several years now.
However, the initial fears that GenAI would take over the role of experts soon dissipated, and expert networks around the world now employ AI to gain a competitive advantage over competitors.
How do they do that, and what does the AI-powered future look like for expert networks? Let’s delve into this topic and consider the main trends of the human-AI symbiosis in expert networks.
Do expert networks engage AI in their processes?
The short answer is ‘yes’. An increasing number of expert networks are using AI to improve their efficiency and accuracy when it comes to sourcing experts throughout various industries.
Before the first large language models appeared on the market, expert networks relied on traditional, static expert databases. This was a constraint that limited the pool of experts and therefore restricted the range of insights accessible to companies engaging an expert network.
Today, numerous expert networks rely on AI to generate real-time data to identify and put their clients in touch with the most suitable subject-matter experts, including those who remain beyond the reach of traditional databases.
These advances in AI are partly the result of significant investments by companies and governments.
AI market statistics in brief
While it is difficult to estimate the global value of the AI market, influential data providers have suggested an astonishing value of US$233 billion.
By 2032, providing there are no disruptions, this is expected to hit US$1,770 billion, with a 29% compound annual growth rate.
The AI market in the United States is expected to be the largest in the world in 2025, with a projected value of US$66 billion, according to Statista.
Experts from Markets and Markets comment that a myriad of industries, including healthcare, finance, manufacturing, and retail, are adopting new technologies, which in turn increases the demand for AI solutions.
This trend is confirmed by a Deloitte survey that indicated 93% of companies set AI adoption as the number one priority in business planning.
Apps that use large language models are predicted to automate 50% of digital work in 2025.
There are currently over 300 million businesses operating worldwide and almost 67% utilize GenAI products that employ LLMs to operate with human language and create content.
Now, let’s examine how exactly AI impacts the expert network industry today.
1. AI-powered expert sourcing
As mentioned, three years ago virtually every expert network relied on a static expert database, which considerably limited the range of insights accessible to them, whereas today, numerous expert networks rely on AI to generate real-time data to identify and connect their clients with the most suitable subject-matter experts.
2. Improved expert-search and expert-matching
AI algorithms learn continuously, which means that each time an organization performs a search, the client’s preferences are remembered, which, together with engagement patterns, ultimately leads to a higher level of accuracy for expert recommendations.
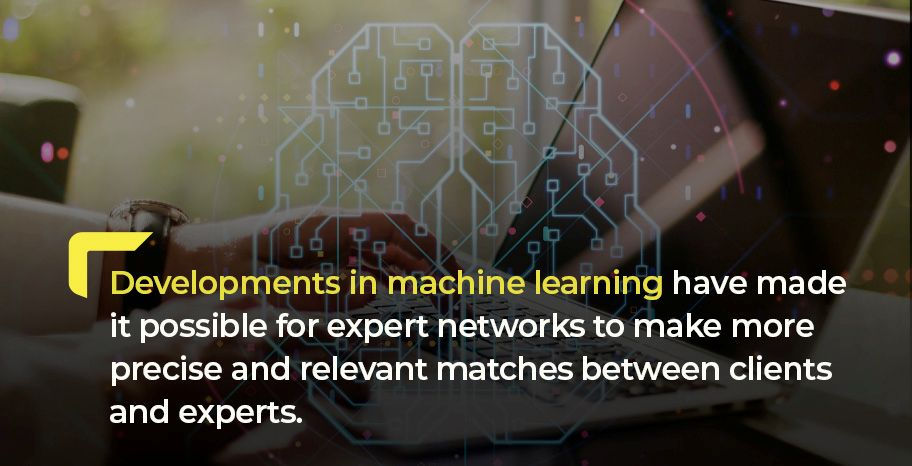
3. Insights automation
AI-powered advanced transcription technologies are used to put the content of expert calls ‘on paper’ and extract key insights, which helps associates to obtain important information in less time.
For instance, using automated segment summaries, companies are free to focus on strategic decisions rather than spending time manually transcribing the information.
4. Knowledge graphs and more structured data
Expert networks employ AI-powered systems to gather and organize information from different sources using knowledge graphs and structured data.
By outlining the connections between various strands of information, these systems help expert networks to find the best expert for each request.
5. Probabilistic modeling for better expert recommendations
With the help of probabilistic models, AI can improve expert recommendations.
Probabilistic models are a type of AI system that is used to predict results based on probabilities (hence the name).
These models do not provide a concrete answer, but examine various patterns and data, take into consideration various possibilities and then generate the best option based on likelihood.
For instance, based on past data and present conditions, a weather prediction model might determine that there is a 70% likelihood of sun, a 20% probability of rain, and a 10% probability of clouds for the following day.
This model is widely used in sectors such as agriculture to determine when to implement plant protection measures against pests and diseases.
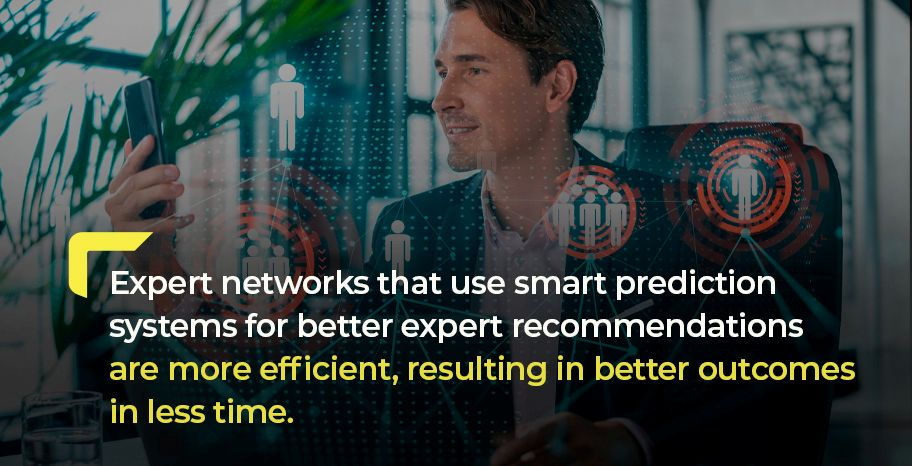
The big question: Can AI models replace human experts?
The short answer (at least for today) is no. But GenAI can complement human experts.
RightAngle clients, for instance, seek access to expert insights that are not available online because they are based on the unique knowledge and experience held by each individual expert.
AI can be a great tool for answering factual questions such as "Which are the top companies in the field of the automotive industry in Germany?" but if deeper insights are required, AI would not be able to help. Human expertise would be needed because some information is simply not publicly available.
However, large language models such as GPT-4 and DeepSeek are effective tools that can rapidly analyze huge amounts of information and extract insightful data, therefore making expert matching much quicker and with greater accuracy.
Another aspect to consider is that AI is far from perfect.
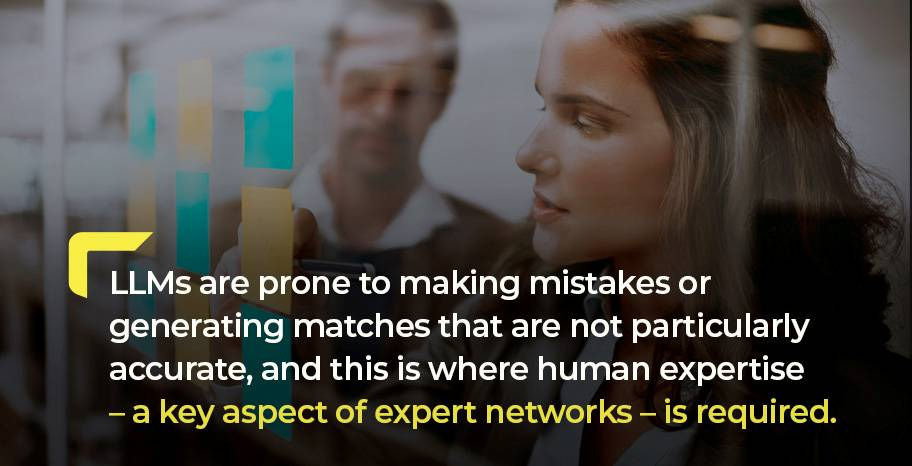
Here are two examples of AI…
Example (1):
If an LLM searches for ‘leading experts in renewable energy’, there is a possibility it could provide information on academics or media commentators when in reality industry practitioners are required, particularly those actively working on new technologies.
If the search is narrowed and the inquiry changed, the system may be unable to locate or track names or suggest any databases where the search could continue.
In this case, associates from expert networks can search for industry experts with hands-on experience who are not online or may not be present on specialized websites. Human reviewers can therefore identify who is actually relevant.
Example 2:
If a client contacts an expert network and wants to learn more about the latest AI regulations in the EU, an LLM can generate only partially correct information.
Because regulations change frequently, it may provide outdated or incomplete information.
Human experts, on the other hand, can be actively involved in regulatory discussions and can search for and offer real-time insights.
A combination of human vetting and AI-powered insights helps expert networks to achieve near-perfect accuracy.
This combination of AI and human judgment could revolutionize the way expert networks operate, leading to more efficient, accurate, and insightful expert recommendations.
The future of expert networks powered by AI could bring groundbreaking improvements to the industry.
Will AI impact the way clients access expert insights?
The short answer is yes, but gradually.
There are a number of tools that help clients to engage with expert networks, including email requests, video calls, transcripts, and surveys, but the whole process of contacting, collecting information, and turning this into meaningful and useful insights is time-consuming. So how can AI help in the future?
One hypothetical scenario is AI replacing expert networks that are operated by human personnel.
It is possible that in the future, clients could engage exclusively with AI, which would summarize all the collected information and deliver insights.
Moreover, it is also possible that clients will engage with virtual AI assistants that look almost human.
Although this is all fairly hypothetical, some changes are already taking place. For instance, we are already witnessing expert networks using AI to generate transcripts and undertake translation, searches, and summarizing.
Is AI adoption really that fast?
In terms of expert networks, the pace of AI adoption is not as fast as some may think even though the technology has been widely and quickly adopted throughout other industries. There are several reasons to explain this:
In AI development there is a specific pattern – firstly there is excitement followed by challenges and eventually gradual improvements which take time.
Expert networks are in need of highly specialized insights, and therefore AI must learn to surf through niche industry knowledge and adapt to each organization’s unique requirements.
AI in expert networks must comply with strict data security and the protection of proprietary information regulations, which means careful planning should be undertaken.
Final word
There is no doubt that AI integration brings a fundamental change to expert networks and the way businesses obtain and employ expert insights.
With the evolution of this technology, expert networks will gradually improve their services, flexibility, accuracy, and efficiency. At the same time, AI will not replace human experts but instead will complement them and in fact already does so.